5G’s claim of ultra-low latency, and suitability for real-time edge processing has created a fever of interest in the IoT market. But what does Real-time dataset analysis really mean for your IoT application?
It’s estimated that the global smart sensor market will have over 50 billion smart devices in 2020. All of these IoT smart sensors (temperature, pressure, gas, image, motion, loadcells) will be connected to Wifi, 5G, LoRa etc network services via embedded processors performing real-time signal processing on the captured datasets.
But there are a number of challenges….
IoT sensor measurement challenge
A common challenge is that many sensors used in these applications require a little bit of filtering in order to clean the measurement data in order to make it useful for analysis.
Let’s have a look at what sensor data really is…. All sensors produce measurement data. These measurement data contain two types of components:
- Wanted components, i.e. information what we want to know
- Unwanted components, measurement noise, 50/60Hz powerline interference, glitches etc – what we don’t want to know
Unwanted components degrade system performance and need to be removed.
So, how do we do it?
DSP means Digital Signal Processing and is a mathematical recipe (algorithm) that can be applied to IoT sensor measurement data in order to clean it and make it useful for analysis.
But that’s not all! DSP algorithms can also help in analysing data, producing more accurate results for decision making with ML (machine learning). They can also improve overall system performance with existing hardware (no need to redesign your hardware – a massive cost saving!), and can reduce the data sent off to the cloud by pre-analysing data and only sending what is necessary.
Do you have a practical example?
All analog sensor signals need to be sampled by a digital system in order to make them usable for analysis in the digital domain. The choice of the sampling frequency is primarily goverend by the maximum frequency that needs to be analysed. But what are design rules?
Consider the following application for gas sensor measurement (see the figure below). The requirement is to determine the amplitude of the noisy sinusoid (shown in blue) in order to get an estimate of gas concentration, where the bigger amplitude, the more the gas concentration.
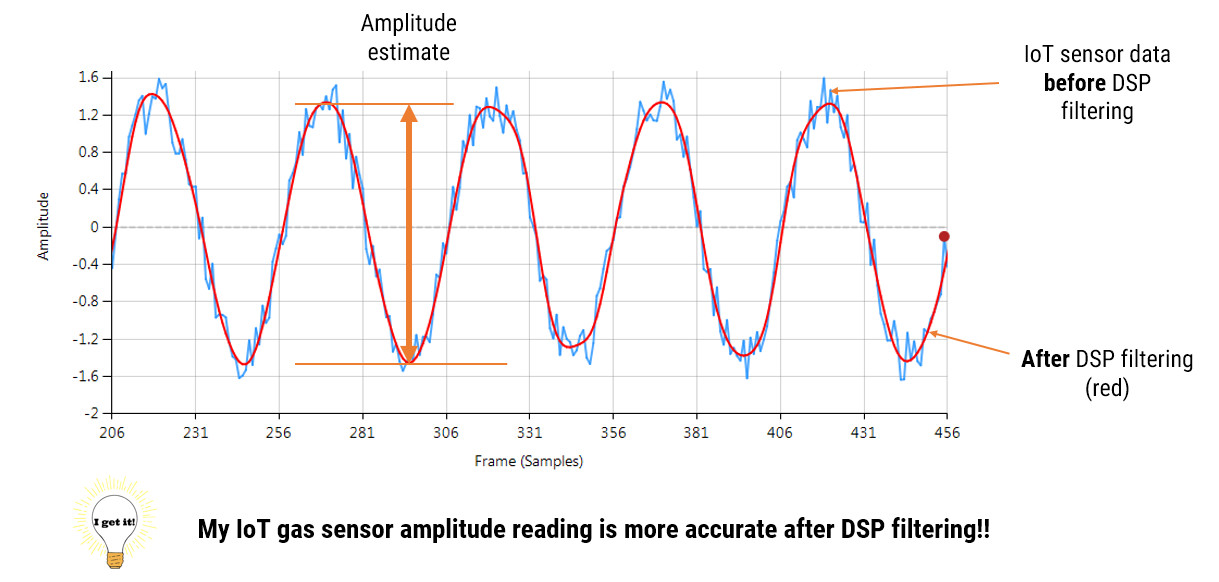
In order to clean the noisy sinusoid with a filtering algorithm (results shown in red), we first need to find what the frequency of the sinusoid is. The Nyquist sampling Theorem is used for determining this value, and states that,
the analog signal must be sampled at a least two times the maximum analog frequency component.
For our gas sensor, the frequency of the blue sinusoid is about 5Hz, so a minimum sampling frequency of 10Hz is required in order to perform valid analysis on the sampled dataset. However, many designers choose a value 10 times higher than Nyquist in order account for the effects of the noise component and not to be on the borderline of the Nyquist-sampling theorem.
The concept of sampling is demonstrated below:
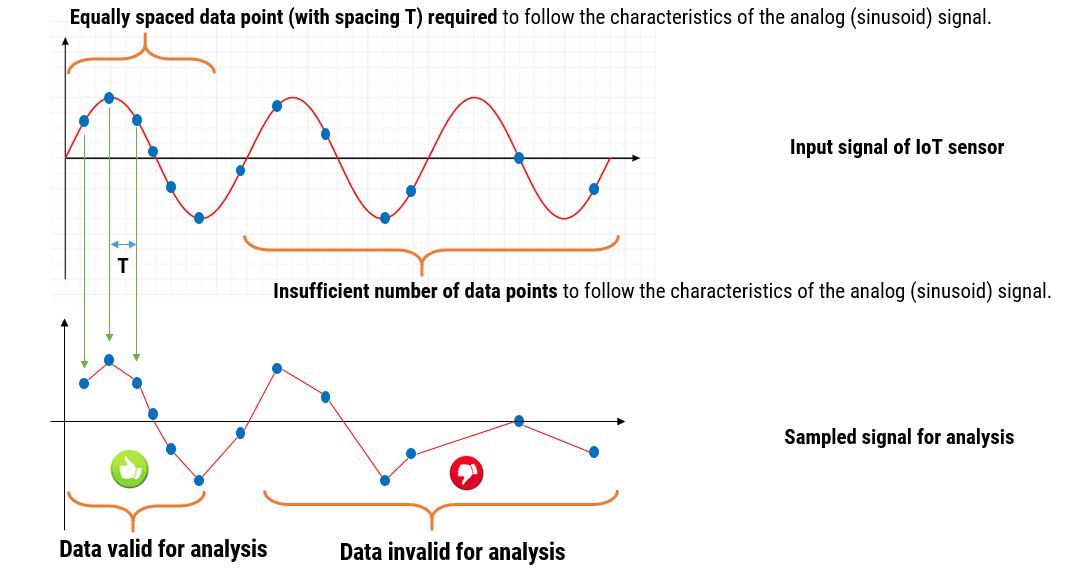
What does Real-time really mean?
Many clients ask us to clarify what real-time really means.
Most people assume that an instant response to a button push or event means real-time. However, the reality is a little more complicated, as a real-time system means that the response is deterministic occurring within a known time frame. This could be seconds or even micro-seconds. In all cases, the response or action time is always known.
For the gas sensor discussed above, the sampling frequency must be constant in order to correctly follow the characteristics of the sinusoid. If the sampling rate varied over time, the sampled data wouldn’t match the design criteria of the algorithmic filtering blocks, and the data analysis would be invalid.
In recent years, much has been said about 5G’s potentially ultra-low latency, and suitability for real-time edge processing. Time will tell how far 5G’s low latency claim can be realised. However, latency in network/cloud services, means that no communication channel can be guaranteed to be real-time 100% of the time. This is further complicated by the requirement of meeting the Nyquist-sampling criteria for sampling analog sensors signals.
In light of all of these issues, our experience has shown that real-time sensor processing (especially for critical automotive or industrial control operations) should be performed at the edge on an embedded real-time processor for maximum reliability and safety.
Our close collaboration with leading technology companies, such as: Arm, Texas Instruments and KPN ensure that our 5G IoT solutions are built with the latest design paradigms using the best of today’s sensor and networking technology.